Note
Go to the end to download the full example code.
Plotting length distributions with fractopo
Initializing
from pprint import pprint
import matplotlib as mpl
import matplotlib.pyplot as plt
# Load kb11_network network from examples/example_data.py
from example_data import KB11_NETWORK
mpl.rcParams["figure.figsize"] = (5, 5)
mpl.rcParams["font.size"] = 8
Plotting length distribution plots of fracture traces and branches
Using Complementary Cumulative Number/Function
# Log-log plot of network trace length distribution
fit, fig, ax = KB11_NETWORK.plot_trace_lengths()
# Use matplotlib helpers to make sure plot fits in the gallery webpage!
# (Not required.)
plt.tight_layout()
plt.show()
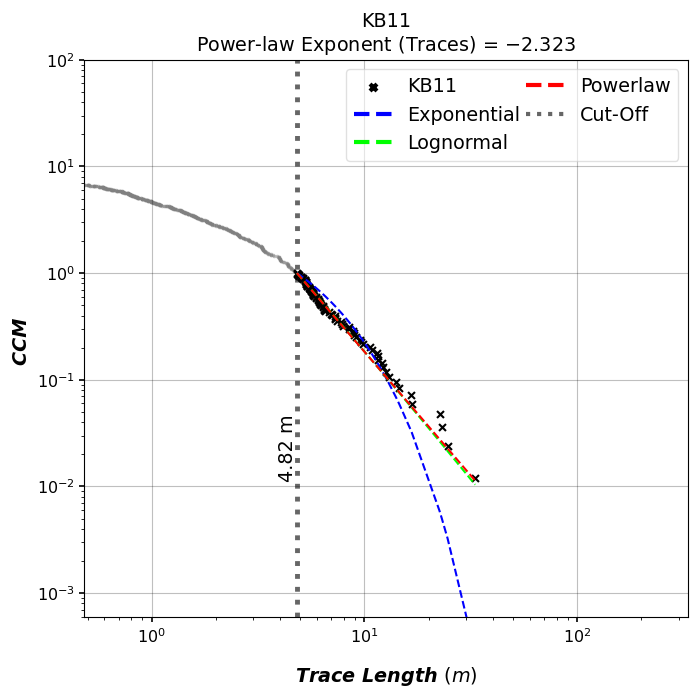
# Log-log plot of network branch length distribution
KB11_NETWORK.plot_branch_lengths()
plt.tight_layout()
plt.show()
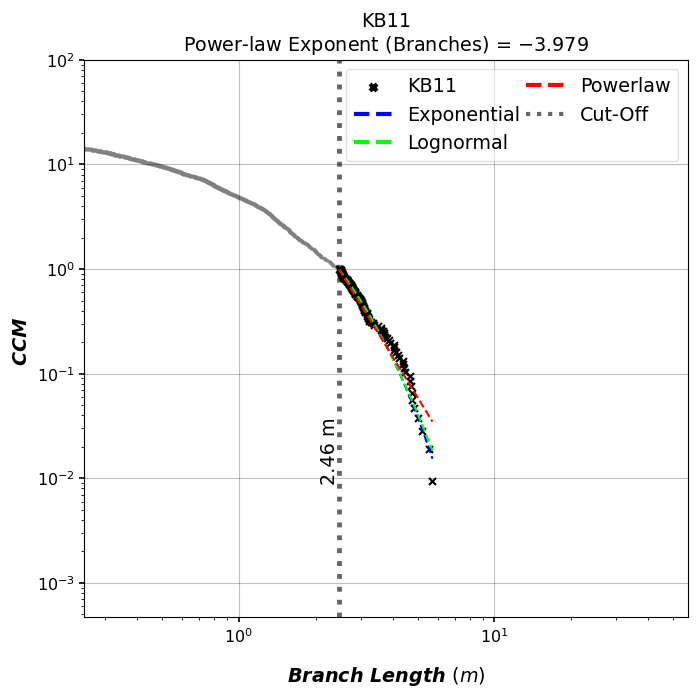
Using Probability Density Function
# Log-log plot of network trace length distribution
KB11_NETWORK.plot_trace_lengths(use_probability_density_function=True)
# Use matplotlib helpers to make sure plot fits in the gallery webpage!
# (Not required.)
plt.tight_layout()
plt.show()
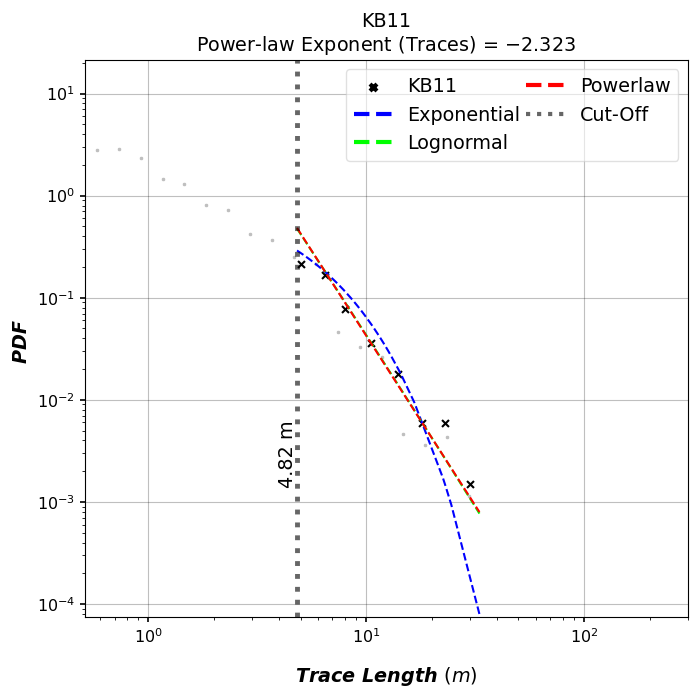
# Log-log plot of network branch length distribution
KB11_NETWORK.plot_branch_lengths(use_probability_density_function=True)
plt.tight_layout()
plt.show()
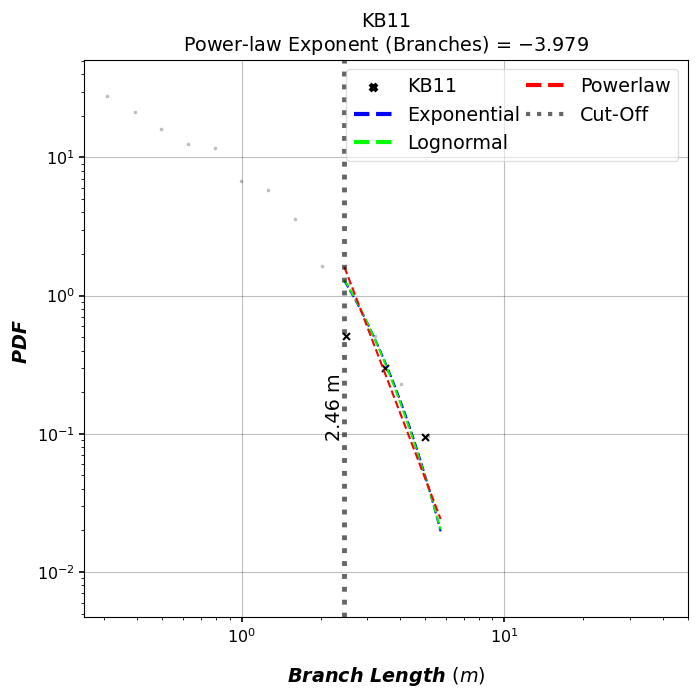
Numerical descriptions of fits are accessible as properties
# Use pprint for printing with prettier output
pprint(KB11_NETWORK.trace_lengths_powerlaw_fit_description)
{'trace exponential Kolmogorov-Smirnov distance D': 0.16734893835952386,
'trace exponential lambda': 0.2898919999912063,
'trace exponential loglikelihood': -188.02077841990499,
'trace lengths cut off proportion': 0.8815232722143864,
'trace lognormal Kolmogorov-Smirnov distance D': 0.055285689798228566,
'trace lognormal loglikelihood': -181.36559959113396,
'trace lognormal mu': -24.654367132184213,
'trace lognormal sigma': 3.413632605089442,
'trace lognormal vs. exponential R': 1.8402477911397208,
'trace lognormal vs. exponential p': 0.06573186649520259,
'trace power_law Kolmogorov-Smirnov distance D': 0.05261149958762154,
'trace power_law alpha': 3.323399316187791,
'trace power_law cut-off': 4.815579557192599,
'trace power_law exponent': -2.323399316187791,
'trace power_law sigma': 0.25350364847712353,
'trace power_law vs. exponential R': 1.7923908641663917,
'trace power_law vs. exponential p': 0.0730703771992165,
'trace power_law vs. lognormal R': -0.0997396742302772,
'trace power_law vs. lognormal p': 0.9205510020886896,
'trace power_law vs. truncated_power_law R': -0.3949163478172344,
'trace power_law vs. truncated_power_law p': 0.6549247892396857,
'trace truncated_power_law Kolmogorov-Smirnov distance D': 0.06088951719124519,
'trace truncated_power_law alpha': 3.101655682133221,
'trace truncated_power_law exponent': -2.101655682133221,
'trace truncated_power_law lambda': 0.01693723427355317,
'trace truncated_power_law loglikelihood': -181.27535493663555}
pprint(KB11_NETWORK.branch_lengths_powerlaw_fit_description)
{'branch exponential Kolmogorov-Smirnov distance D': 0.055610337970091184,
'branch exponential lambda': 1.2799623045206825,
'branch exponential loglikelihood': -79.83654595014787,
'branch lengths cut off proportion': 0.9488416988416989,
'branch lognormal Kolmogorov-Smirnov distance D': 0.056242712153426244,
'branch lognormal loglikelihood': -80.11030644664194,
'branch lognormal mu': 0.3954002513747114,
'branch lognormal sigma': 0.48573668994587976,
'branch lognormal vs. exponential R': -1.2860976878833073,
'branch lognormal vs. exponential p': 0.19840897095369647,
'branch power_law Kolmogorov-Smirnov distance D': 0.05150595395106583,
'branch power_law alpha': 4.9790958462418935,
'branch power_law cut-off': 2.4602426467400713,
'branch power_law exponent': -3.9790958462418935,
'branch power_law sigma': 0.38648395404192654,
'branch power_law vs. exponential R': -1.2796975009064637,
'branch power_law vs. exponential p': 0.20065154388298556,
'branch power_law vs. lognormal R': -1.2484090815914302,
'branch power_law vs. lognormal p': 0.21188128491686808,
'branch power_law vs. truncated_power_law R': -1.56450118561549,
'branch power_law vs. truncated_power_law p': 0.05525806104829145,
'branch truncated_power_law Kolmogorov-Smirnov distance D': 0.05467641228173603,
'branch truncated_power_law alpha': 1.0000206602209936,
'branch truncated_power_law exponent': -2.066022099356246e-05,
'branch truncated_power_law lambda': 1.0171370451351636,
'branch truncated_power_law loglikelihood': -79.8378452140172}
Set-wise length distribution plotting
pprint(KB11_NETWORK.azimuth_set_names)
pprint(KB11_NETWORK.azimuth_set_ranges)
('N-S', 'E-W')
((135, 45), (45, 135))
fits, figs, axes = KB11_NETWORK.plot_trace_azimuth_set_lengths()
Total running time of the script: (0 minutes 3.560 seconds)